Vocabulary Word of the Day: Probability
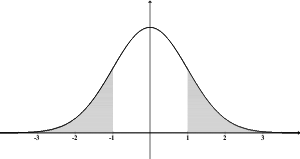
I know headline writers need their splashy headlines, but as the media is filled with word of a stunning upset, we should remember the number of times that one candidate or the other was “destroyed” or “finished,” or the election was declared “over.”
A poll takes a snapshot of part of the electorate which is extrapolated to the whole. It will have a margin of error, generally something like 2.5% to 3.5%. (Why that is can be a project for research.) That means that a candidate who is at 50% in that poll might actually be as low as 46.5% and as high as 53.5% if the error margin is 3.5%. If we’re thinking of two candidates, the other, let’s say showing at 45%, could be anywhere from 41.5% to 48.5%, an overlap of 2%. Now that’s using a margin for candidate A of +5%. The average for Clinton was around +3.5% the day before the election. Now not all polls were at that value, and also not all polls have a 3.5% error margin.
Now there’s an additional percentage involved, which is a probability, variously 90-95%, that the poll itself is within that margin. So in the poll above, if the figure is 95%, for example, 95 out of 100 times the election being polled would reflect a result within that margin for error. Otherwise it might be anywhere. I’m not making any effort here to keep these details realistic — if you read up on the topic you can learn how these numbers relate, but more importantly, if you read the data on a poll, you can find what these numbers are for that particular poll.
There is no set way to combine polls into an aggregate, and there is no established error margin for polls that are combined. That’s because not all polls are created equal. On the eve of the election, Nate Silver and crew were giving something near a one in four chance that Trump would win the election, i.e., according to their analysis, if you had a good enough sample, one in four elections run where the data looked like this would go to Trump. Three in four would go to Clinton. The election doesn’t make them wrong. That’s a probability.
Let’s look at that. If I flip a coin, the probability is one in two that it will come up heads an one in two that it will come up tails. So I flip the coin, and it comes up heads. Was my projection wrong? Not at all. Similarly, the FiveThirtyEight people aren’t wrong either. If they had said, “Clinton will be the next president of the United States,” then they would have been wrong. What they said was that there was around a 78% chance it would be Clinton an a 22% chance it would be Trump.
They were critiqued by Sam Wang of the Princeton Election Consortium, and several people wrote on FiveThirtyEight to defend their methodology. Dr. Wang gave a 99% chance that it would be Clinton. Both Nate Silver (and an unknown number of members of his crew) and Dr. Wang are much more skilled at this than I am (in the same sense that an MLB player is more skilled at baseball than I, considering I have never picked up a baseball bat for a game, is better at baseball than I am). I spent some time with their data and couldn’t really find a way to understand fully how they got their probabilities and why they differed so much. (On your mental flowchart, create a box and label it “Lots of Statistical Figuring.”) But my intuitive feeling was that Nate Silver was getting the better of the argument. It seemed to me that there was insufficient data on which to base that high a level of confidence in the aggregation of these polls. We haven’t been polling presidential elections for that long.
Why don’t we have neat numbers for the aggregate values? Let me note, first, that any time one uses a phrase like “the polls show,” one is doing some sort of aggregation, however loose. Folks like Sam Wang and Nate Silver do it in a very scientific way. (I’ll let them argue over which is more scientific, and they do so with some vigor.) We all do it when we look at polls and make a generalization. The reason there isn’t a neat x% margin of error an y% probability that the poll will, in fact, fall within that margin is that polls use different methodologies. If you average the number of apples an oranges you have, you don’t get a better value each for apples an oranges. It might be better to say that if I average my Gala apples an my Golden Delicious apples, I don’t get an accurate picture of what type of apples I have available. One set of those apples Jody wants to bake into a crisp, and the other I’m going to slice up and eat. I’m afraid I’ll have to look at the actual apples.
Again, my inexpert intuition is that aggregation needs more experience and testing to get more accurate, even as I think that Nate Silver’s work is the more promising. In the meantime, what is quite certain is that nobody in the media has a clue about any of this. Alternatively, they don’t care, and just want to write headlines to sell papers, whether they reflect actual data or not. I suppose that’s possible.